Expected results
COMMON DATA MODEL
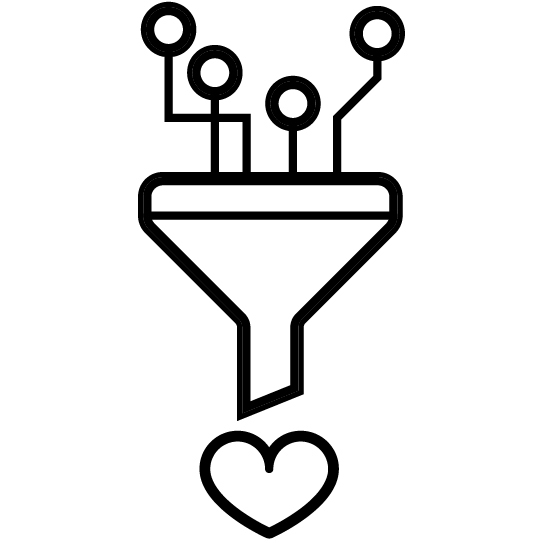
To improve quality, interoperability, machine-readability, and re-use of health data and metadata in line with the Findability, Accessibility, Interoperability, and Reusability (FAIR) data management principles, and exploit multisource and large-scale data in cardiology, DataTools4Heart will deliver a Common Data Model (CDM) based on the international, open-source health data exchange standards (Health Level 7 in Fast Healthcare Interoperability Resources – HL7 FHIR). CDM will be designed with different characteristics (linguistic features, diagnostic and referral patterns from Electronic Health Records – EHRs), as a standardised set of Common Data Elements (CDEs) of medical, surgical, and pharmacological records to ensure structural and semantic interoperability across heterogeneous data sources, multiple Machine Learning (ML) models, and Virtual Assistants (VAs).
METADATA CATALOGUE
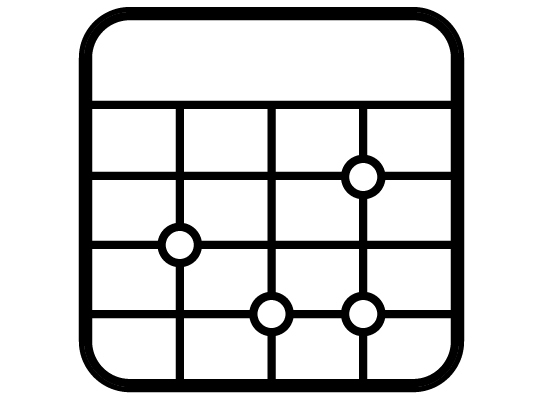
A DataTools4Heart Metadata Catalogue will be developed to ensure that information on data sets of the Common Data Model (CDM) distributed over sites is ‘findable’ in Findability, Accessibility, Interoperability, and Reusability (FAIR) terms via provenance (lineage and processing history) and governance (ownership, usage rules, and metrics). Such a catalogue will be able to display aggregated statistics and build queries on top of the CDM, using clinical concepts as well as through medical terminology systems. Thus, datasets based on the statistics of clinical datasets i.e., containing sex, cardiovascular risk assessment, and lab tests results, will be enabled. The Metadata Catalogue will be built upon the guidelines of the European Open Science Cloud (EOSC) Dataset Minimum Information (EDMI) and the data catalogue of the euCanSHare project.
MULTILINGUAL NATURAL LANGUAGE PROCESSING SUITE
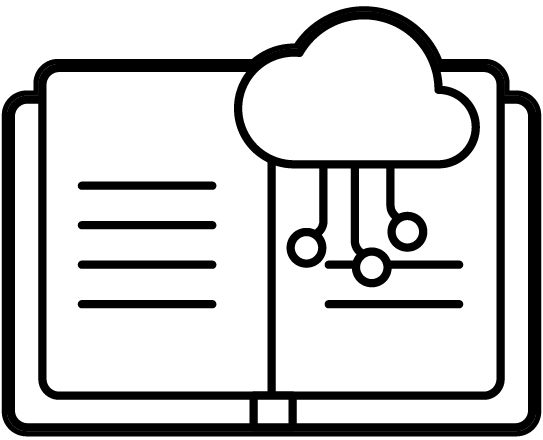
DataTools4Heart will introduce a multilingual Natural Language Processing (NLP) suite, including cardiology-specific entity recognition and machine translation, for standardised data structuring of cardiology reports across European regions. NLP will use 7 language (English, Spanish, Italian, Romanian, Czech, Swedish, and Dutch) models to be adapted to the cardiology domain, using Electronic Health Records (EHR). Technical analysis will include coverage, semantic interoperability, and cross-language adaptation of at least 4 general medical ontologies (ICD10, SNOMED-CT, RxNorm, and UMLS) and 3 cardiology-specific ontologies (ACA/AHA EHR, CTO, and CDO) used for clinical applications.
FEDERATED LEARNING
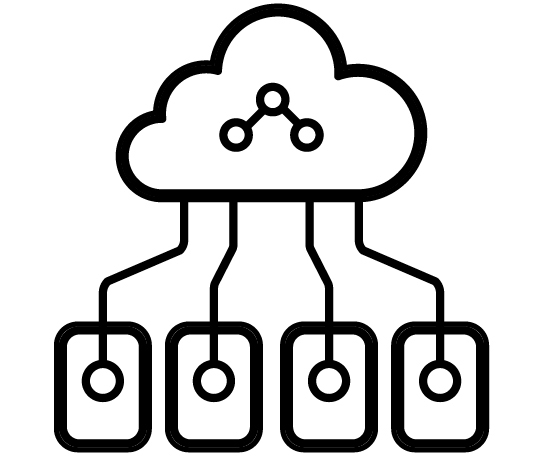
Federated Learning (FL) allows Artificial Intelligence (AI) models to be trained across multiple edge devices or centres, without data movement. Preliminary results with FL have been encouraging in Oncology, Neurology, and Covid-19. In cardiology, Consortium partner University of Barcelona obtained promising results on multi-centre cardiac image analysis. DataTools4Heart will introduce centre dropout and uncertainty-aware aggregation to create a novel FL pipeline characterised by increased fairness and efficiency.
DIFFERENTIAL PRIVACY
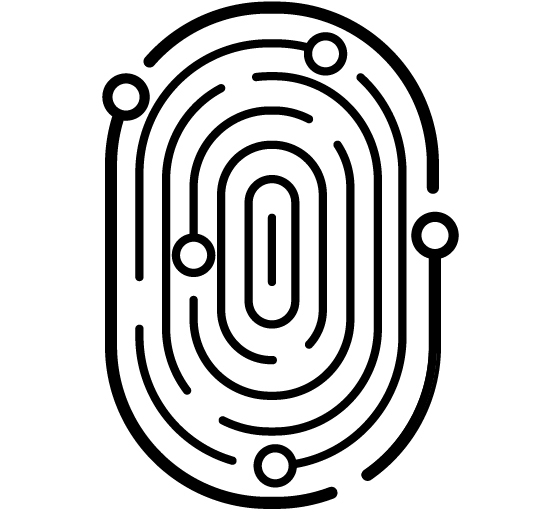
DataTools4Heart will focus on pseudonymous data within the legal framework of the General Data Protection Regulation (GDPR), leveraging a Federated Learning (FL) system, and differentially private synthetic data as an innovative form of anonymous data. DataTools4Heart’s technical toolkit will provide a data ingestion and Natural Language Processing (NLP) framework which will work within a FL platform. Multilingual processing and standardisation of clinical free text or unstructured records augment the type and volume of structured data that is available and re-usable across borders. DataTools4Heart leverages relevant European projects for the re-use of health data to assess contributions and limitations. Two sets of European projects have been identified to this end.
SYNTHETIC DATA GENERATION
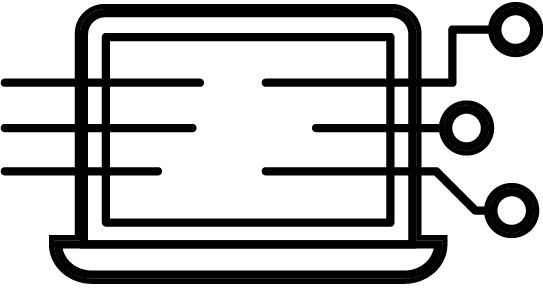
DataTools4Heart will propose new robust methods of Real-World Evidence (RWE) collection and data synthesis. Differentially private synthetic data will act as an innovative form of anonymous data with the advantages of being representative of the target population, able to expand its size whereas necessary, sharable for research and increasing knowledge, and usable to reduce bias in algorithmic development. As a result of this effort the CardioSynth dataset will be created as a private by design synthetic dataset which will remain as open-source legacy for further research. DataTools4Heart will validate the synthetic data generation through a comparative study of models learnt from synthetic data vs other means (privacy-preserving and not).
ARTIFICIAL INTELLIGENCE - VIRTUAL ASSISTANTS
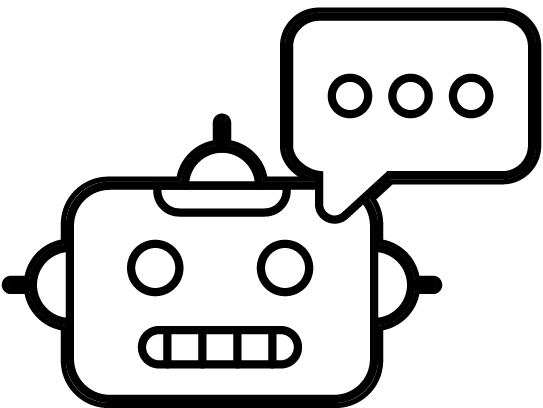
DataTools4Heart will develop an open platform with Artificial Intelligence-powered Virtual Assistants (AI-VA), as a main user interface, to support multilingual interaction in at least 7 languages and help clinicians, researchers and data scientists navigate through large-scale multi-source federated patient datasets in clinical cardiology. AI-VA usability will be demonstrated and assessed through pilot studies based on concrete real-world clinical use-cases for heart disease patient referral in outpatient and emergency units, with wide validation across 7 hospitals across Europe. AI-VA will be built to facilitate use of the DataTools4Heart tools, including visualisation capabilities and uncertainty estimates that will be rigorously tested.
CARDIOSYNTH DATASET
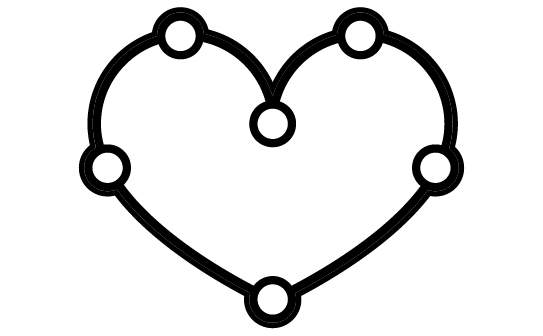
DataTools4Heart will generate and make available CardioSynth, a synthetic dataset for cardiology with clinically consistent structured and unstructured data, working as a federated, General Data Protection Regulation (GDPR) compliant, open-source resource for the research and professional community. The CardioSynth Dataset will be produced and made openly available to be used to fine-tune the concept normalisation system and find eligible groups of synthetic patients.
CODE OF CONDUCT
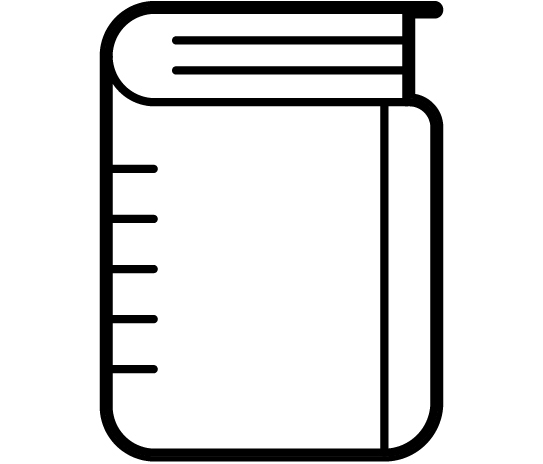
DataTools4Heart will propose a draft “Code of Conduct” to ensure compliance with the General Data Protection Regulation (GDPR), including legal and technical standards for the responsible reuse and sharing of pseudonymized and synthetic health data across EU Member States. The Code will be informed by input from DT4H events with stakeholders and patient advocacy groups and will provide a standard for clinical research entities, whether private or public, as data controllers or processors. The aim is to facilitate secure sharing of pseudonymized and synthetic data in the medical research field, while preserving the rights of the data subjects.